AI Tool Predicts Pre-Diabetes, Diabetes in High-Risk Patients
Researchers have found that an artificial intelligence-based tool can predict pre-diabetes and type 2 diabetes in populations at high risk for the conditions.
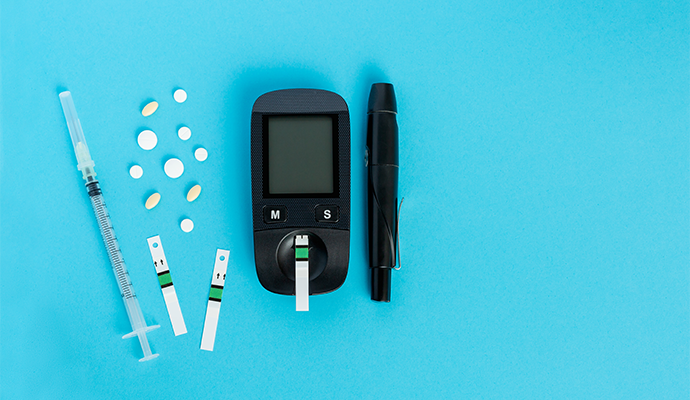
Source: Getty Images
- New research published in BMJ Innovations last week shows that a machine-learning (ML) algorithm trained to analyze electrocardiogram readings can accurately predict pre-diabetes and type 2 diabetes in at-risk populations, which have the potential to advance diabetes screening in the future.
According to the CDC’s most recent National Diabetes Statistics Report, both pre-diabetes and diabetes are a major population health concern in the US, despite the incidence of newly diagnosed diabetes dropping from 9.3 per 1,000 US adults in 2009 to 5.9 per 1,000 in 2019. Currently, it is estimated that 96 million Americans over the age of 18, or 38 percent of the adult US population, has pre-diabetes and 11.3 percent of the US population has diabetes.
Similar trends can be seen internationally as well, making diabetes a major focus of chronic disease research worldwide. In this study, researchers looked at participants from the Diabetes in Sindhi Families in Nagpur (DISFIN) study, which examined the genetic basis of type 2 diabetes in families at high risk for the disease.
The study authors note that previous research in this area has shown that ECGs can provide early insights into the presence of pre-diabetes and diabetes sooner, before other clinical signs related to blood glucose and hemoglobin. ECG-based methods are also less invasive than standard diagnostics for the two conditions, which could improve accessibility of diabetes screening if clinically validated, the researchers state.
For this study, families with at least one known case of type 2 diabetes were enrolled. Participants were asked to provide their personal and family medical histories, along with details about their diet, prior to any testing. Then, all participants underwent a range of blood tests and clinical assessments to help detect pre-diabetes and diabetes using American Diabetes Association criteria.
Just over 1,200 people were included in the study, and each had a standard ECG performed as part of their clinical assessment. From these, 100 structural and functional features from each lead were used to generate the predictors for the algorithm used in the study, known as DiaBeats.
Over 10,000 heartbeats were recorded from participants, which were split into training, validation, and independent test sets. The algorithm was tasked with classifying each participant as “no diabetes,” pre-diabetes, or type 2 diabetes based on ECG inputs.
Overall, diabetes prevalence in the cohort was high: 14 percent had pre-diabetes and 30 percent had type 2 diabetes. The ML algorithm achieved high performance in accurately identifying these participants, detecting the presence of the two conditions with accuracy and precision of 97 percent, independent of other factors like age, gender, and other metabolic disorders.
These findings, according to the researchers, indicate that their model may provide a more accessible alternative to current diabetes diagnostic methods to detect these diseases earlier, if clinically validated.
Other AI methods have also been used to stratify diabetes risk.
In April, researchers from the National Institutes of Health (NIH) Clinical Center developed a model capable of detecting type 2 diabetes risk using CT images that showed factors related to pancreatic health and fat levels.
AI has also been used to improve diabetes management, as shown in 2019 research from the Rensselaer Polytechnic Institute. As part of the research, information from thousands of continuous glucose monitors and insulin pumps were collected and evaluated based on performance and how often faults occur. These insights would then be used to improve the devices.