Artificial Intelligence Approach Helps Identify Type 2 Diabetes Risk
A new artificial intelligence method used various factors, including pancreas health and fat levels, to determine patients at risk for developing type 2 diabetes.
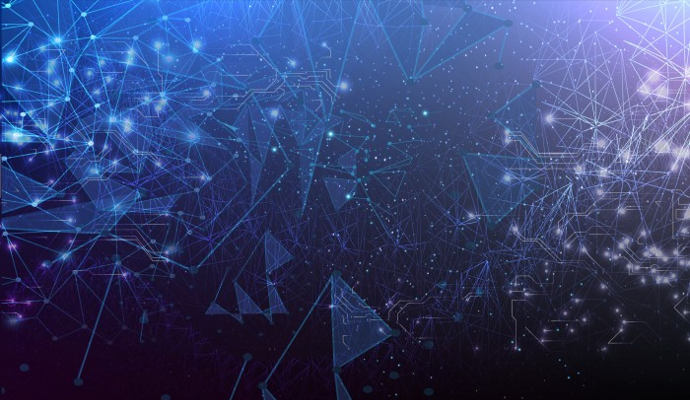
Source: Getty Images
- Researchers from the National Institutes of Health Clinical Center developed a new artificial intelligence (AI) model that analyzed various factors relating to pancreas health and fat levels using non-contrast abdominal CT images to detect type 2 diabetes risk.
The study, which was published in Radiology, evaluated 8,992 patients, of which 572 had type 2 diabetes mellitus, and 1,880 had dysglycemia. All patient screenings occurred between 2004 and 2016.
To build the model researchers used 471 images obtained from various datasets. They divided the photos into three categories: 424 for training, 8 for validation, and 39 for test sets.
The researchers used a variety of measurements when analyzing images. These measurements included CT attenuation, pancreatic volume, intrapancreatic fat percentage, and three-dimensional fractal dimension.
Other analyzed features included visceral fat levels and density and volumes of neighboring muscles and organs.
Results showed that low pancreas density and high visceral fat correlated with diabetes prevalence.
The consistent and accurate results displayed by the AI model indicated that fully automated analyses of abdominal CT biomarkers are a reliable approach to predicting and diagnosing diabetes.
Further, researchers hope to continue implementing multivariable analyses in diagnosing type 2 diabetes.
“This study is a step towards the wider use of automated methods to address clinical challenges,” said study authors Ronald M. Summers, MD, PhD, and Hima Tallam, an MD and PhD student, in a press release. “It may also inform future work investigating the reason for pancreatic changes that occur in patients with diabetes.” Summers and Tallam work and study at the National Institutes of Health Clinical Center, respectively.
The authors did note various limitations of the research, including difficulty segmenting images, and the exclusion of clinical factors such as race, family history, or blood lipid level from the final model.
Increasingly, predictive analytics methods are being used in clinical diagnoses.
For example, a predictive analytics method developed in August 2020 used a calculator to help clinicians foresee a stroke. Specifically, the tool used information regarding the metabolic system, including blood pressure and cholesterol.
Another study published in Nature Communications described how an early-warning system monitored infectious diseases. The study involved 3,000 providers who reported data on influenza-like illnesses to the Centers for Disease Control and Prevention weekly. With this data, the researchers created a forecasting model that intended to provide early warnings regarding patients at risk for stroke.
But as predictive analytics use in healthcare evolves, researchers are taking steps to enhance these methods.
Research published in the Journal of Social Computing in June 2021 described how predictive analytics could best succeed when assisted by both AI and humans, as AI works well when handling big data, and humans are better at handling thick data.