Artificial Intelligence Diagnoses Adverse Childhood Experiences
Using real-time data and explainable artificial intelligence, researchers developed a system that could aid clinicians in diagnosing and treating adverse childhood experiences.
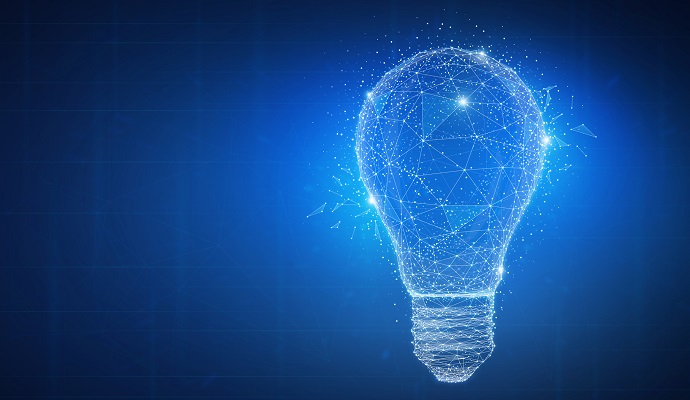
Source: Getty Images
- An explainable artificial intelligence platform that analyzes data captured during in-person consultations may enhance diagnosis and treatment of adverse childhood experiences (ACEs), according to a study published in JMIR – Medical Informatics.
ACEs are negative events and processes that an individual might encounter during childhood or adolescence. These events have been proven to be linked to increased risk of a range of negative health outcomes and conditions in adulthood.
Because the social determinants of health have a similarly profound impact on physical well-being, researchers noted that many studies have focused on studying the links between social determinants of health, ACEs, and health outcomes.
However, there are few intelligent tools available to assist in the real-time screening of patients and assess the connection between ACEs and social determinants of health, which could help guide patients and families to available resources.
The research team worked to develop an AI platform that could help providers diagnose ACEs in the early stages.
"Current treatment options are long, complex, costly, and most of the time a non-transparent process," said Arash Shaban-Nejad, PhD, MPH, an assistant professor at the Center for Biomedical Informatics in the Department of Pediatrics at the University of Tennessee Health Science Center.
"We aim not only to assist healthcare practitioners in extracting, processing, and analyzing the information, but also to inform and empower patients to actively participate in their own healthcare decision-making process in close cooperation with medical and healthcare professionals."
When designing the platform, the group aimed to develop an algorithm that could incorporate context into its predictions and decisions. Many AI tools don’t provide enough explanation to interpret and justify decisions, which can prolong the diagnosis and treatment process.
“Although a standard AI algorithm can learn useful rules from a training set, it also tends to learn other unnecessary, nongeneralizable rules, which may lead to a lack of consistency, transparency, and generalizability,” the team noted.
“Explainable AI is an emerging approach for promoting credibility, accountability, and trust in mission-critical areas such as medicine by combining ML techniques with explanatory techniques that explicitly show why a recommendation is made.”
Researchers implemented an AI platform called Semantic Platform for Adverse Childhood Experiences Surveillance (SPACES), which analyzes real-time data captured through conversations during in-person consultations.
“The idea behind the SPACES platform is to monitor the causes of ACEs and social determinants of health, and their impacts on health,” the group stated.
“This platform can provide the contextual knowledge needed to facilitate intelligent exploratory and explanatory analysis. Through this framework, decision makers can (1) identify risk factors, (2) integrate and validate ACEs and social determinants of health exposure at individual and population levels, (3) and detect high-risk groups.”
To make real-time recommendations, the system instantly captures new resource interests, ACEs, and social determinants of health-related risk factors detected in the user’s current conversation and uses them to incrementally refine a personalized knowledge paragraph.
At each stage in the conversation, a question-answering agent passes detected entity types and contextual parameter values to the recommendation service. Entity types help the service determine entry points on the knowledge graph, and contextual parameters help refine the queries further to obtain a more personalized version of the graph.
The team’s prototype is intended for several types of users, including caregivers and healthcare professionals. The main features of the platform that would be available to healthcare professionals are recommendations for digital assistance, studying the association between ACEs and social determinants of health, knowledge graph querying, and geocoded resource recommendation.
Researchers expect that their proposed approach could help providers diagnose and treat ACEs, and better understand the context surrounding the technology’s decisions and predictions.
“The significance of the proposed approach lies in its ability to provide recommendations to the question-answering agent with the least effort from both the user and the healthcare practitioner,” researchers said.
“It aims to maximize knowledge about the patient without having to delve into all of the questions that are often asked in ACEs and social determinants of health intake assessments. It also provides the ability to explain why a certain question or resource was suggested.”