Data Questions Sepsis Prediction Models, Predictive Analytics
Researchers at Michigan Medicine suggest that Epic’s sepsis prediction model is less accurate than previously claimed.
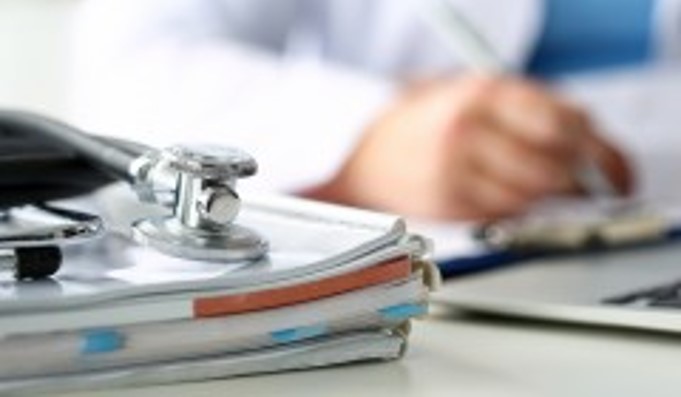
Source: Getty Images
- In a recent evaluation of Epic Systems’ sepsis prediction model, scientists suggest that the model identifies those at risk of sepsis just 63 percent of the time. This is much lower than the model’s information sheet claims at between 76 and 83 percent, the researchers said.
This comes as 56 percent of hospitals and health systems in the United States use Epic Systems.
Sepsis presents itself as a challenging issue in healthcare. According to the Centers for Disease Control and Prevention (CDC), one and three patients who die in the hospital have sepsis, but is difficult to predict which patients are at risk of developing the condition.
“Sepsis is something we can know occurs with certainty after the fact, but when it’s unfolding, it’s often unclear whether a patient has sepsis or not,” Karandeep Singh, MD, MMSc, assistant professor of Learning Health Sciences and Internal Medicine at Michigan Medicine, said in a report. “But the cornerstone of sepsis treatment is timely recognition and timely therapy.”
According to Singh, the problems with Epic’s model are found in how it was developed. The model collected data from all cases that were billed as sepsis. Singh explained that “people bill differently across services and hospitals and it’s been well recognized that trying to figure out who has sepsis based on billing codes alone is probably not accurate.”
Additionally, in the model’s development, the onset of sepsis was defined as the time the physician intervened. “In essence, they developed the model to predict sepsis that was recognized by clinicians at the time it was recognized by clinicians. However, we know that clinicians miss sepsis,” Singh said.
When working with predictive models, healthcare providers must make a tradeoff. “The tradeoff is basically between generating alerts on a patient who turned out not to have the predicted condition or not generating alerts on patients who do,” Singh stated.
The study done by Singh and his team concludes that there is a need for more regulatory oversight and governance of clinical software tools. Additionally, models should be open-sourced and able to easily turn off if they are not useful.
While their analyses differed, Singh said Epic’s was not necessarily wrong. “We differ in our definition of the onset and timing of sepsis. In our view, their definition of sepsis based on billing codes alone is imprecise and not the one that is clinically meaningful to a health system or to patients,” Singh said.
In response to the report, a spokesperson from Epic Systems said, “Clinicians often will recognize many of the patients who are septic. The purpose of the model is to identify early the harder to recognize patients who otherwise might have been missed. It does this by indicating the probability of a likelihood of sepsis. The model has been shown to identify patients who are coming down with sepsis up to 4 hours earlier than clinicians identify them, and that can be lifesaving.”
“In the example given in this paper, if the Epic model was used in real time it would likely have identified 183 patients who otherwise might have been missed. The author’s study did not take into account the analysis and required fine tuning that needs to occur prior to real-world deployment of the model. They also picked a threshold value which casts a wide net to include more patients as possibly septic, yielding more false positives. Customers have complete transparency into the sepsis model,” the spokesperson continued.
“The full mathematical formula and model inputs are available to administrators on their systems. Accuracy measurements and information on model training are also on Epic’s UserWeb, which is available to our customers.”