Machine Learning May Support Personalized Mental Health Therapies
Using machine learning, researchers detected variability among children’s neural anatomy that could inform personalized treatments for mental health disorders.
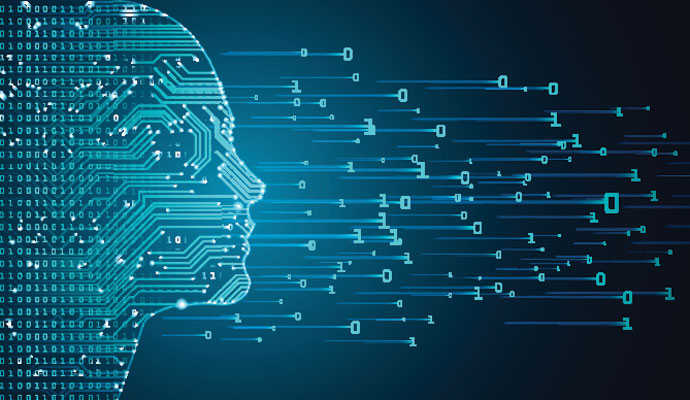
Source: Getty Images
- Researchers from Penn Medicine leveraged machine learning techniques to identify the size and shape of brain networks in children, which could lead to improved understanding and more personalized treatment of mental health conditions.
Published in the journal Neuron, the study used machine learning tools to analyze the functional magnetic reasoning imaging (fMRI) scans of nearly 700 children, adolescents, and young adults. The analysis is the first to show that neuroanatomy can vary significantly among children, which is refined during development.
The human brain has a pattern of folds and ridges on its surface that provide physical landmarks for finding brain areas. To study the functional networks that govern cognition, researchers will typically line up activation patterns with these physical landmarks. However, this process assumes that the functions of the brain are located on the same landmarks in each person.
Multiple studies of adults have shown that this isn’t the case for more complex brain systems responsible for executive function, a set of mental processes that include self-control and attention.
In these systems, the functional networks don’t always line up with the brain’s physical landmarks of folds and ridges – instead, each adult has their own specific layout. Until this study, researchers didn’t know how these person-specific networks might change as kids grow up or relate to executive function.
“The exciting part of this work is that we are now able to identify the spatial layout of these functional networks in individual kids, rather than looking at everyone using the same ‘one size fits all’ approach,” said senior author Theodore D. Satterthwaite, MD, an assistant professor of Psychiatry in the Perelman School of Medicine at the University of Pennsylvania.
“Like adults, we found that functional neuroanatomy varies quite a lot among different kids—each child has a unique pattern. Also like adults, the networks that vary the most between kids are the same executive networks responsible for regulating the sorts of behaviors that can often land adolescents in hot water, like risk taking and impulsivity.”
The team analyzed a sample of 693 adolescents and young adults between ages eight and 23. The participants completed 27 minutes of fMRI scanning as part of the Philadelphia Neurodevelopment Cohort (PNC), a large study funded by the National Institute of Mental Health.
Using machine learning algorithms, researchers mapped 17 functional networks in individual children, rather than relying on the average location of these networks. The group then analyzed how these functional networks evolved throughout adolescence and were related to performance on a series of cognitive tests.
The results showed that the functional neuroanatomy of these networks was refined with age, and helped the researchers predict the age of a child with a high level of accuracy.
“The spatial layout of these networks predicted how good kids were at executive tasks,” said Zaixu Cui, PhD, a post-doctoral fellow in Satterthwaite’s lab and the paper’s first author. “Kids who have more ‘real estate’ on their cortex devoted to networks responsible for executive function in fact performed better on these complex tasks.”
The study offers new insights on developmental plasticity and diversity, and could pave the way for more personalized diagnostics and therapeutics.
“The findings lead us to interesting questions regarding the developmental biology of how these networks are formed, and also offer potential for personalizing neuromodulatory treatments, such as brain stimulation for depression or attention problems,” said Satterthwaite.
“How are these systems laid down in the first place? Can we get a better response for our patients if we use neuromodulation that is targeted using their own personal networks? Focusing on the unique features of each person’s brain may provide an important way forward.”