New ML Models Use Brain Activity Patterns to Track Seizures’ Origins
New research describes how two machine-learning tools developed by Johns Hopkins researchers can determine where seizures begin and the success of surgeries with 79 percent accuracy.
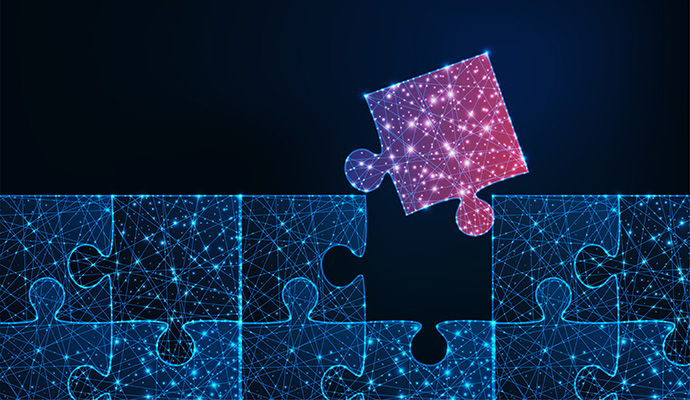
Source: Getty Images
- As described in the journal Brain, a set of machine-learning (ML) tools developed by Johns Hopkins University researchers displayed the ability to accurately determine brain activity that may lead to seizures, ultimately improving epilepsy treatment.
According to the Centers for Disease Control and Prevention (CDC), 1.2 percent of the US population had active epilepsy in 2015. Epilepsy is a brain disorder that causes seizures, affecting people in various adverse ways.
A press release from Johns Hopkins also describes how 30 percent of people with epilepsy are drug-resistant, resulting in only two treatment options: a device that is implemented into the brain to stop seizures or a surgery that involves the removal of brain regions that control seizures. However, this type of surgery is only effective about half the time, according to the press release.
The two new ML models presented in the study displayed the ability to find where seizures originate, enabling clinicians to focus treatment on that area of the brain.
"If you find that zone and you effectively treat it, it's a game changer—it's a life-changing treatment for these patients," said Sridevi V. Sarma, PhD, associate director of Johns Hopkins Institute of Computational Medicine and head of the Neuromedical Control Systems Lab, in a press release.
The models use equations based on machine learning and calculus to determine brain activity patterns, thereby identifying the originating sites of seizures.
"This is a new paradigm," said Joon-Yi Kang, MD, a neurologist at Johns Hopkins Hospital who co-authored the studies, in the press release. "We're getting more insights into specific brain networks. We're not waiting around for seizures to happen."
The research team led by Sarma studied patients' brains when they weren’t having seizures and when their brains received a quick electrical pulse stimulation.
In the models, the brain is a network of nodes that influence each other. The team hypothesized that the absence of a seizure is due to the nodes in the part of the brain where seizures begin being constrained by nodes in the healthy area of the brain. However, during a seizure, the nodes swap roles. The researchers were able to identify the strength and direction of the nodes, leading to the identification of where seizures originated.
Of the 65 patients studied, researchers found that the models could predict the onset of seizures and the success of surgery with 79 percent accuracy.
This research adds to growing evidence showing how artificial intelligence (AI) can be applied in epilepsy care.
A study published in Soft Computing in April described an AI model that classified seizures more accurately than standard methods. Researchers integrated a convolutional neural network architecture with a hierarchical attention mechanism to develop the AI model.
Comprising a feature extraction layer, a hierarchical attention layer, and a classification layer, the model analyzes a feature map from a raw electroencephalography (EEG) signal to determine whether the EEGs it derives from are healthy or indicate the possibility of seizure.