Pathology Foundation Model Leverages Medical Twitter Images, Comments
Stanford researchers have developed a public pathology dataset and a foundation AI model based on diagnostic images and comments from medical Twitter.
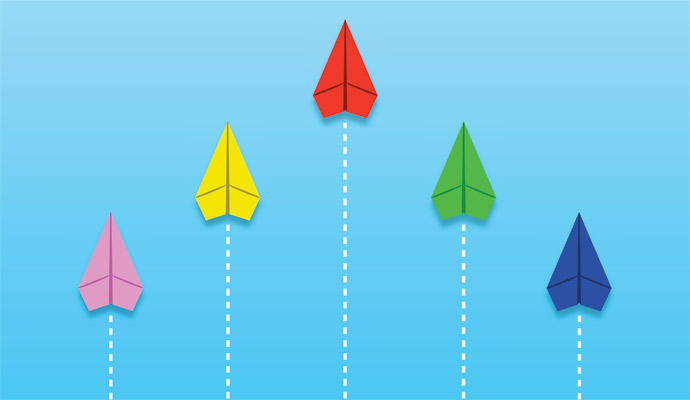
Source: Getty Images
- Researchers from Stanford have leveraged pathology images and comments from Twitter, now known as X, to develop a large public dataset and artificial intelligence (AI) model for pathology image classification and clinical decision support.
The work, detailed in a study published last month in Nature Medicine, posits that a lack of high-quality, publicly available medical images is a significant hurdle to innovation in pathology. However, resources like Twitter communities can help bridge this gap.
“One of the biggest challenges of developing diagnostic AI is lack of large-scale annotated data,” explained James Zou, a professor of biomedical data science and member of the Stanford Institute for Human-Centered AI (HAI), in a news release. “But right there was this highly trained group of physicians sharing data and insights on social media. Medical Twitter is a tremendous boon to medical AI.”
The study indicates that the United States and Canadian Academy for Pathology (USCAP) and the Pathology Hashtag Ontology projects recommend approximately 32 Twitter pathology subspecialty-specific hashtags, which users on medical Twitter use in combination with images and comments to effectively ‘label’ de-identified pathology data.
“A pathologist would run up against something they’d never seen before and post the image to the community, asking, ‘What do you think is going on here?’ And a knowledgeable group of colleagues around the world would respond in written text,” Zou said. “In that combination of text and images, we had our resource.”
Using over 243,000 diagnostic images, their comments, and other data from medical Twitter from 2006 to 2022, in addition to 32,000 annotated images from public datasets, the research team developed OpenPath, a large public pathology dataset with natural language descriptions.
The researchers then utilized OpenPath to train Pathology Language-Image Pre-training (PLIP), a foundation AI model.
The tool can understand text and images, allowing it to be used like a pathology-specific Google Image search, according to a Stanford Medicine blog post. For example, a provider could input an image or text description into the tool to search for similar annotated images or to match a new image to a handful of relevant disease descriptions based on the image’s features.
To evaluate PLIP’s ability to classify data it has never seen before, the researchers tested it using four external validation datasets and compared its results to that of an existing pathology image classification model known as CLIP.
Across the four datasets, PLIP achieved an F1 score of 0.6 to 0.8, whereas the CLIP model achieved scores of 0.3 to 0.6.
“[The model’s] pairing of images and text makes it quite useful. PLIP enables researchers to retrieve similar cases by searching using either images or words,” Zou said. “We think it should greatly facilitate knowledge sharing within the pathology community worldwide.”
The researchers also concluded that the study demonstrates the potential for publicly shared medical information—such as the data found on medical Twitter and other social media platforms—to help advance biomedical AI.
Other studies have also highlighted how AI may play a role in pathology moving forward.
In 2022, researchers from Harvard Medical School and Brigham and Women’s Hospital showed that a deep learning tool could learn pathology image features and detect similar cases.
The tool, called Self-Supervised Image Search for Histology (SISH), is designed to act as a pathology-specific search engine to help clinicians across multiple use cases including rare condition identification.
SISH demonstrated that it could improve the diagnosis and treatment process for these rare conditions.