Risk Prediction Model Accurately Identifies Women Vulnerable to HIV
New model flags recent pregnancy, recent hepatitis C diagnosis, and substance use among risk factors that can identify women most at risk for HIV infection.
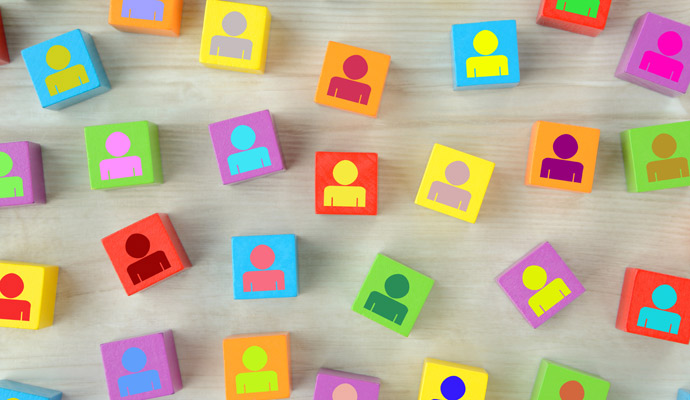
Source: Getty Images
- Researchers from the University of Chicago and Rush University Medical Center have developed a predictive model designed to identify women vulnerable to human immunodeficiency virus (HIV) using EMRs and demographic data, according to a study published recently in BMC Women’s Health.
The authors noted that several models have been created to predict individuals at the highest risk for HIV. However, many of these tools rely on data from all persons newly diagnosed with HIV, the majority of whom are men who have sex with men (MSM).
The authors indicated that risk factors flagged by these predictive models, as a result, are biased toward features and behaviors that only apply to men in general or MSM. Recent HIV trends suggest an overall decline in new infections, but experts underscore that these drops in HIV rates are predominantly attributed to White MSM.
Individuals in other racial, ethnic, age, and sex and gender groups experience varying rates of HIV incidence and associated health disparities. One of these groups is women, particularly Black women, the authors posited.
This led the researchers to create a predictive model for women to identify those at high risk of HIV who may benefit from clinical prevention measures.
To build the tool, the research team matched 48 newly diagnosed women to 192 HIV-negative women based on number of previous encounters at University of Chicago or Rush University hospitals. Both hospitals have large opt-out HIV screening programs.
For each participant in the cohort, data for the two years prior to either their last clinical encounter or their HIV diagnosis were assessed. Using these data, the researchers evaluated clinical risk factors and demographic information from EMRs.
The resulting multivariable logistic regression model’s predictive performance was then tested and measured using area under the curve (AUC).
The research team found that multiple clinical diagnoses were significantly associated with HIV in the cohort, and included these in the model: pregnancy, hepatitis C, substance use, and sexually transmitted infections (STIs), specifically chlamydia, gonorrhea, and syphilis.
Race, ethnicity, and age group were included a priori in the model due to the known increased risk of HIV among certain demographic groups.
The final model included healthcare site, age group, race, ethnicity, pregnancy, hepatitis C, substance use, and STI diagnosis as risk factors, achieving an AUC of 0.74.
The researchers concluded that this demonstrated the model’s acceptable discrimination between those who were and were not newly diagnosed with HIV. The risk factors identified in this study could be incorporated by other health systems to help detect women most at risk for HIV and who may benefit from preventive interventions like pre-exposure prophylaxis (PrEP).
The development of HIV risk prediction models is only one approach to tackle HIV among many, including post-exposure prophylaxis (PEP), antivirals, telehealth-based treatment, and newer tools still being researched, such as gene therapies or an HIV vaccine.
However, issues like racial health disparities in PrEP access and medication adherence challenges have encouraged increased research into preventing HIV infection and spread first and foremost.
In March, UPMC leadership detailed how the health system would be exploring the use of machine learning (ML) to personalize and enhance HIV testing.
Many new infections are transmitted by individuals who aren’t aware that they have HIV, making testing and informing patients of their status critical to UPMC’s HIV prevention strategy. However, the expertise required to effectively leverage the multiple types of HIV tests and the potential for false-positive and false-negative test results hampers these efforts.
To address this, researchers created an ML model designed to enable personalized workflows for HIV screening results based on their classification as likely true- or false-positive. The tool may help improve care for UPMC’s low-prevalence and high-risk patient populations.