Scalable Tools Assisted Domestic Violence Risk Assessment
According to new research, prediction tools supported decision-making in treatment allocation for health services related to domestic violence.
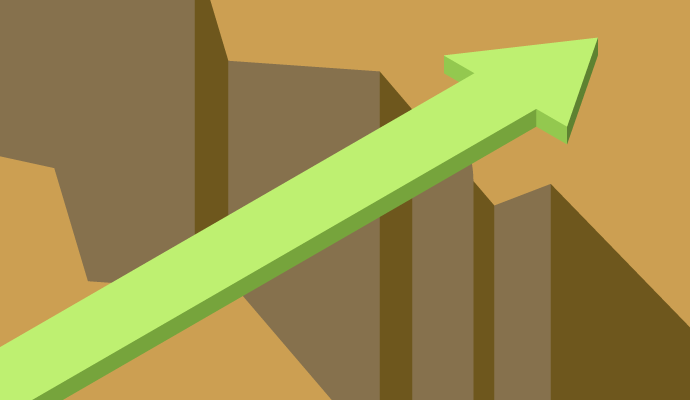
Source: Getty Images
- A new study effectively detects those at the highest risk of domestic violence re-offenses. This data would be valuable in allocating treatment toward malleable risk factors among high-risk patients.
According to the study, about one-third of women and girls experience domestic violence at least once. Published in JAMA Network Open, the study also indicated the wide prevalence ranges, which include 16 percent in Central Europe, 25 percent in North America, and 44 percent in Central sub-Saharan Africa.
Although there are existing risk assessment tools for domestic violence t, many are inaccurate. To counter this issue, researchers created new tools to determine the likelihood of re-offense. They directed these resources toward people who previously perpetrated domestic violence between 1998 and 2013 in Sweden. The study cohort included 27,456 people, with a mean age of 39.4. Approximately 90.3 percent of the population were men.
The models focused on three re-offending outcomes following an arrest for domestic violence. These included the conviction of a new violent crime, any new offense, and re-arrest for domestic violence at the one, three, and five-year points. The creation of models took place under the consideration of sociodemographic, criminological, and mental health details.
Of the total study cohort, 15.4 percent were domestic violence re-offenders who also faced convictions of violent crime. This took place during a mean follow-up of 26.5 months. Meanwhile, 32.8 percent were re-offenders who faced convictions of a new crime. This took place during a mean follow-up of 22.4 months. They also found that 7.6 percent faced rearrest for domestic violence during a mean follow-up of 25.7 months.
These results indicated that prediction tools are scalable and can support decision-making. They would be instrumental in the arrest stage, as they could assist in determining the risk of re-offense. These resources could also benefit the allocation of treatment by allowing criminal justice services to direct attention toward modifiable risk factors.
Although they may vary in terms of structure, risk prediction tools can be applied to various circumstances.
In April, for example, Nationwide Children’s Hospital created and applied a machine-learning model that considered the deterioration risk index to predict pediatric deterioration.
According to research, the speed of recognition of high-risk patients is critical to the treatment process due to the rapid pace of patient deterioration.
In this effort, researchers used a machine-learning model to perform this task faster than traditional methods.
"Predictive algorithms focused on improving clinical care have been increasingly developed over the years, but the vast majority are not operationalized," Laura Rust, MD, emergency medicine physician, physician informaticist at Nationwide Children's, and lead author of the paper, said in a press release. "Transitioning the algorithm from the computer to the bedside can be a long process and requires engagement and collaboration from clinicians, data scientists, and clinical informaticists. This project has been a 5-plus year journey, and we are really proud of the successful integration within our safety culture and the impact on patient outcomes."
These studies are integral examples of how risk assessment methods are vital to treatment. As research shows, data and potential outcome awareness are critical and can greatly affect the health system and patients.